Latest News
AI and International Arbitration: Technical, Ethical, and Legal Implications
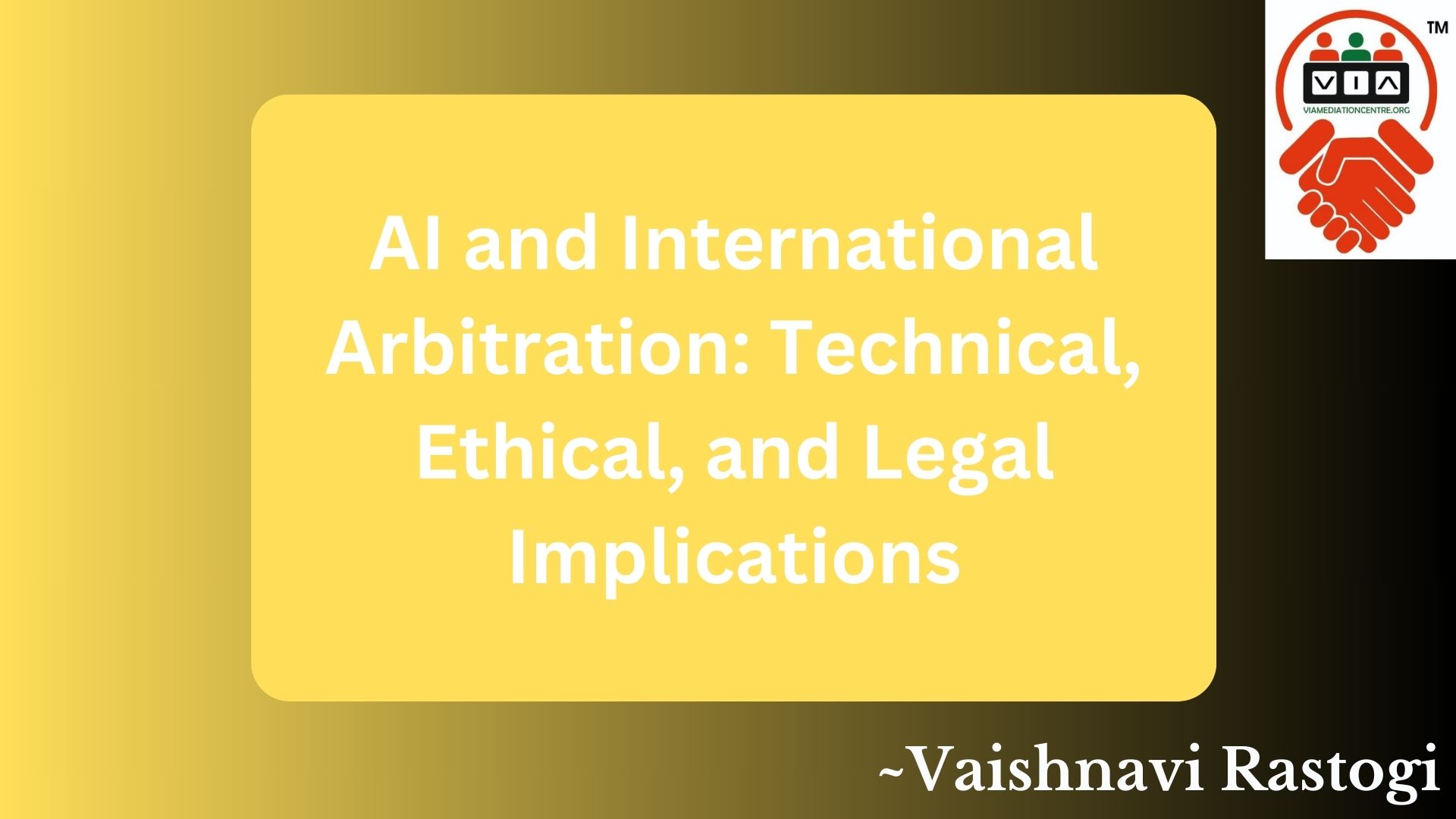
AI and International Arbitration: Technical, Ethical, and Legal Implications
Legal decision-making might be greatly impacted by artificial intelligence (AI), especially in the context of international arbitration. Much research hasn't been done on the effects of using AI, yet some claim that it will always exist and that there should be scepticism since emotional justice and empathy are important. This article looks at the technical aspects of artificial intelligence (AI) and its applications, constraints, and effects on human decision-making. Making a machine operate in a way that a person would be deemed intelligent is known as artificial intelligence (AI). The current body of research on the use of AI to predict court decisions is evaluated critically, casting scepticism on the technology's widespread applicability. Based on the four Vs of Big Data, the paper also examines the drawbacks of AI models, including the requirement for non-confidential case data, recurrent fact patterns, policy modifications, and worries about bias and data diet vulnerability. From a theoretical perspective, the effects of AI decisions on legal theories of judicial decision-making hint at a major paradigm change.
The area of machine learning in artificial intelligence is concerned with computer systems that can learn from their mistakes and keep becoming better at what they do. To increase performance, it entails deducing hidden aspects or patterns from visible data by using vast volumes of data and processing capacity. Machine learning has applications in many domains, including legal decision-making and language learning. For instance, a 2016 academic study focused on textual data taken from judgments and looked at decisions made by the European Court of Human Rights over three articles of the European Convention on Human Rights. Based on the supposition that the text's predictive performance of the Court's decision outcome aligns with other empirical work on judicial decision-making in difficult cases and supports fundamental legal realist intuitions, the study's authors suggest that their findings may open the door for ex-ante prediction of future ECtHR cases.
Ex ante outcome prediction in US Supreme Court decisions is feasible, as the research shows. It is unclear, nonetheless, if the operative part and the Court's legal reasoning from the ECtHR judgments were taken into account while contributing to the study. Since future cases cannot be predicted due to the unknown ex-ante reasoning of the Court, the study's total prediction rate of 79% must be taken into account. Few input characteristics are specific to the issue, however, the research employed over 28,000 case results and over 240,000 individual justices' votes as input data. The decision outcome prediction is restricted to binary classification problems when the task involves predicting whether the Supreme Court will uphold or overturn a decision made by a lower court. The results of the study raise concerns about whether artificial intelligence models should support or replace human decision-makers.
The foundation of data-driven efforts is comprised of the four Vs of Big Data: Volume, Variety, Velocity, and Veracity. They highlight the challenges posed by using Big Data and aid in the assessment of data-driven AI algorithms in the legal industry. The four Vs stand for volume, variety, velocity, and veracity and represent the limits of artificial intelligence-based data-driven models for legal decision-making. The most important prerequisite for a data-driven AI program is volume since machine learning models are data-hungry and need a large sample size to forecast results with any degree of accuracy. AI models perform better in domains where there is a large number of decisions made on a particular topic. The second problem is data intake, specifically whether AI-based models for decision-making can handle complex and non-repetitive topics. When it comes to dealing with a variety of distinct and varied challenges, international commercial arbitration is less likely to adopt AI systems than international investment arbitration. The model's output presents the third difficulty as it might be impacted by shifts in policy and the number of available data. AI models are probably going to stick to 'conservative' techniques that are in line with earlier instances.
Accuracy and reliability of data are referred to as veracity, particularly when discussing artificial intelligence (AI). Compared to humans, AI models are infallible and have the advantage of algorithmic impartiality, but they are susceptible to the effect of non-rational, subjective factors. Because the underlying data used to train the algorithm may have been tainted by preconceptions held by humans, data diet vulnerability might result in systemic errors. This may cause the model to become biased and make inaccurate predictions.
The explainability or interpretability of AI research outcomes is a difficulty. Since AI models frequently rely on pattern recognition rather than specified rules, it can be challenging to explain how they generate results. To overcome these challenges, researchers in AI are creating Explainable Artificial Intelligence (XAI), which compares outcomes and identifies the reasons behind variations using counterfactual scenarios. However, because statistical or probabilistic models have limits, it is difficult to give explanations or arguments for AI decision-making. Thus, by addressing the difficulties of striking a balance between algorithmic impartiality and the complexity of human decision-making, AI models have the potential to completely transform legal decision-making.
Judges should make decisions based only on logic and logical reasoning, with the law functioning as a self-contained system. AI techniques, like machine learning models, could defy this formalist interpretation of judicial reasoning, nevertheless. Neural nets are examples of machine learning models that frequently employ probability rather than logic and lack explicit rules. This might not follow the legal syllogism and instead run counter to it. Legal realists contend that judges apply reasoning to mechanically apply established norms to create law. Beyond the concepts of legal realism, probabilities are frequently used by AI models as a normative foundation. Legal theories that deal with judicial decision-making ought to make a distinction between their normative or prescriptive component and their descriptive component. It would not only be against legal formalism but also beyond the ideals of legal realists to replace logical, deductive, and rule-based reasoning as the normative basis for judicial decision-making with probabilistic judgments.
By: Vaishnavi Rastogi
- AI in legal decision-making faces challenges with data volume, variety, velocity, and veracity.
- AI models show promise in predicting court decisions but are limited to binary outcomes and may not account for complex legal reasoning.
- AI models risk bias from flawed data and lack transparency, complicating their application in judicial contexts.